[03/2023] Do we have a best friend, a god or just a tool?
On being sustainable, fair, equanimous, while listening to (good?) music
How do we know when we are doing the right thing? This could very much be a Barbie vs Oppenheimer question, and you would be right to deem it a Barbenheimer since in the end, a fair and equanimous society in both cases looked like something to strive for and fight for, and for sure not a given.
In this issue we talk about the ethical issues and sustainability of AI, which will become more and more relevant as we go on about in our daily life regardless of what we are aware of right now, as well as the importance of the language we use for it.
As with every new technological milestone we try to make sure to keep an eye also on the possible dangers, such as the bias of facial software recognitions towards black women in particular. We also keep an eye of what the music majors are about to do with the rights they achieved from artists, from the moment the next user generated content will also include deepfakes based on their voices. Also, this might be both a great news or its opposite, we are not far from AI being able to guess what music we have been listening to. Can we still have secrets if we want to?
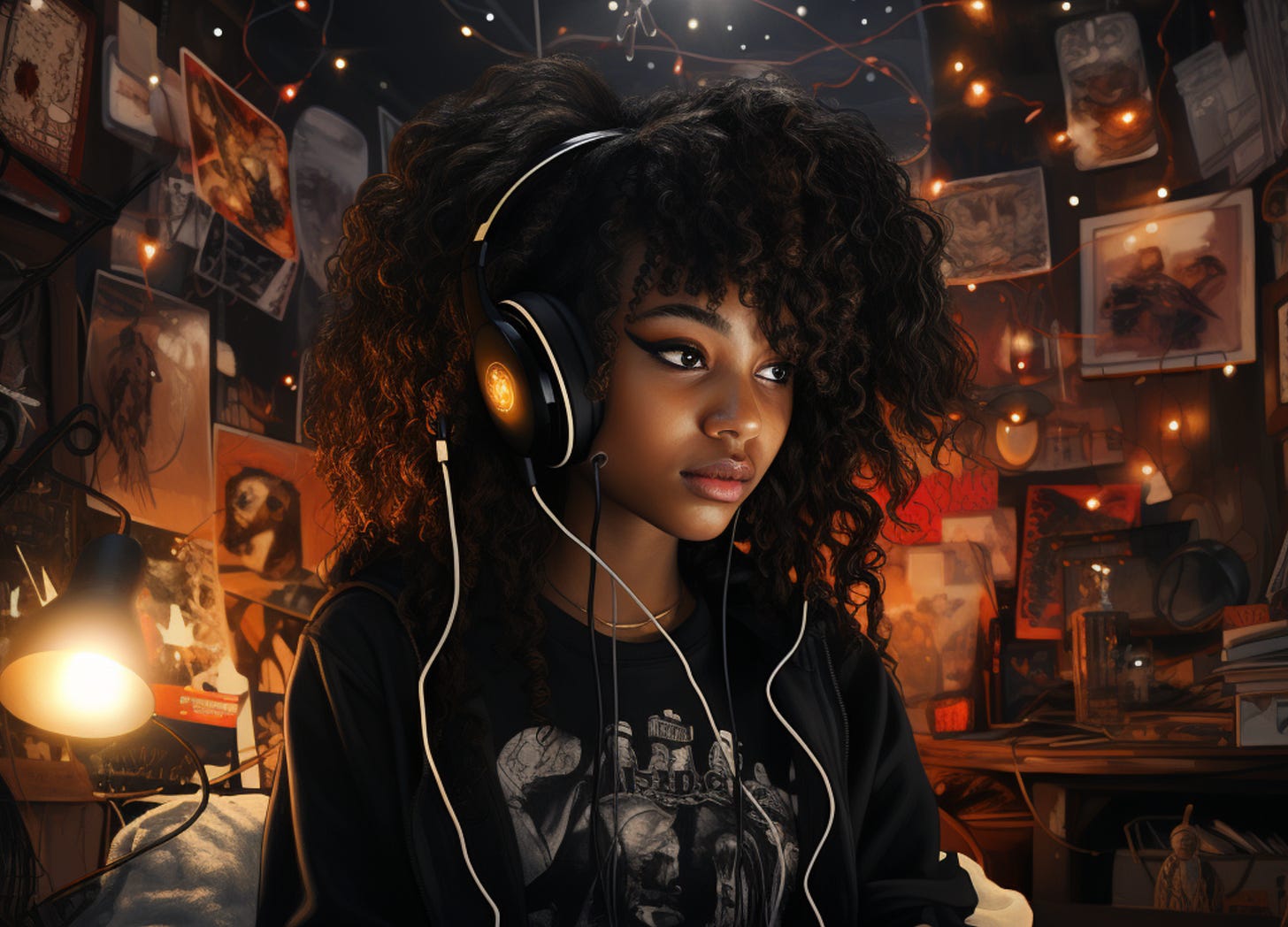
On being tone deaf, creating music, and trying to guess which song you listened to through AI
To be 8 months pregnant, woman and black
This is one of the many stories where police were looking for a thief. The victim had been scammed by a woman who, after having had a sexual encounter with him, left with his car and his wallet. Police ran a software to match people in the area with mugshots and 6 people were identified as the possible culprit. The victim, who was also the eyewitness in this case, recognised a person among those selected by the AI software: it was a black eight months pregnant woman, and she was innocent. It took her a month to prove it.
She is, as of this week, the sixth person being falsely accused of a crime as a result of facial recognition technology used by police to match an unknown offender’s face to a photo in a database. All six people were black, and we know that algorithms are 10 to 100 times more likely to inaccurately identify a photograph of a black or East Asian face, compared with a white one. In searching a database to find a given face, most of software pick incorrect images among black women at significantly higher rates than they did among other demographics.
In our previous issue we spoke about the unreliability of AI medical chatbots, riddled with notorious problems such as hallucinations and biases to mention some. At the same time we stressed the concrete risk that their trustworthy human-like communication style could lead to an increase in the perception of credibility of the answers given, regardless of their unreliability. A similar problem is rising in AI-based facial recognition software, used experimentally in US to corroborate eyewitness evidence.
Eyewitness, although universally used, is not a reliable evidence as such because our memory is often inaccurate and because we are intuitively led to believe that confidence equals reliability (of the eyewitnesses’ memories). Results are unfair and horrible. There have been instances when somebody has been recognised as the perpetrator of a crime by an eyewitness and, after years of conviction, had been proved innocent by DNA. Similarly in this case, both AI and the eyewitness blamed an innocent person.
What is being done to prevent that AI becomes a tool for criminalisation of minorities in the future? According to this report we have for now just a mere, simple, laconic hypothesis:
the quality of the data could improve the AI performance. [emphasis mine]
From the right to stream to the right to deepfake
What do we do when the user generated content includes deepfakes? The question is being answered as we write. DeepFakes of the voices of musical artists will be soon available for everybody to make, at a price. Alphabet/Google and Universal music are in talks to create a licensing system to allow users to create deepfakes using singers’ voices through AI. Warner, the third biggest music major in the world, could follow suit.
Some might remember when the same players, the then Google and the recording majors, sat on the table to negotiate rights to stream music videos in 2009. At the time they created Vevo, a music and video entertainment service best known for providing music videos to YouTube
The service was launched as a joint venture among three major record companies: Universal, Sony and Emi. Warner joined in 2016. The concept behind Vevo was to use its platform to syndicate music videos to YouTube and then share the revenues between Google and the Majors. According to the sources:
Robert Kyncl, chief executive of Warner Music, on Tuesday told investors that “with the right framework in place”, AI could “enable fans to pay their heroes the ultimate compliment through a new level of user-driven content . . . including new cover versions and mash-ups”. He added that artists should have a choice to opt in. “There are some that may not like it, and that’s totally fine,” he said. For Google, creating a music product could help the company compete with rivals such as Microsoft, which has invested $10bn in leading AI company OpenAI, owner of the market-leading AI model known as GPT-4.
Making music from text and guessing what music you just listened to? Done deal
There is some more to say about Alphabet/Google and the musical field, as the company is experimenting more and more with AI in this field. MusicLM is a new machine learning product recently launched by Alphabet/Google that can generate high fidelity audio from text.
This is not the only project in the works. The researchers that created MusicLM went further with experimenting what AI can do with musical samples so that they could develop a method for reconstructing music from brain activity customized for each person, drawing links between their unique brain activity patterns and various musical elements.
According to this article:
After being trained on a selection of data, the AI could convert the remaining, previously unseen, brain imaging data into a form that represented musical elements of the original song clips. The researchers then fed this information into another AI model previously developed by Google, called MusicLM. MusicLM was originally developed to generate music from text descriptions, such as "a calming violin melody backed by a distorted guitar riff."
MusicLM used the information to generate musical clips that can be listened to online and fairly accurately resembled the original song snippets — although the AI captured some features of the original tunes much better than others.
"The agreement, in terms of the mood of the reconstructed music and the original music, was around 60%,
Let's go back to the basics
Is Artificial Intelligence Sustainable?
It's no news that the spread of generative artificial intelligence has a considerable environmental impact. While AI can be an ally in addressing climate change, its daily usage by individuals raises sustainability concerns. How can we tackle this issue? This is the subject of two articles that I suggest you read:
Environmental impact of ubiquitous generative AI (Towards Data Science)
Green Intelligence: Why Data and AI Must Become More Sustainable (Forbes)
An Ethical Compass for AI
In no other field is the ethical compass more relevant than in artificial intelligence. These general-purpose technologies are re-shaping the way we work, interact, and live. The world is set to change at a pace not seen since the deployment of the printing press six centuries ago. AI technology brings major benefits in many areas, but without the ethical guardrails, it risks reproducing real world biases and discrimination, fueling divisions and threatening fundamental human rights and freedoms.
The Recommendation on the Ethics of Artificial Intelligence by UNESCO is not a brief read: published in 2021, it has been adopted by all 193 member states. The interesting aspect is the Policy Action Areas, which make them adaptable to numerous fields of application, from healthcare to the environment, from data governance to research. They are worth a full read.
Do Not Forget the Essential
Last but not least, one aspect worth remembering is that - when discussing artificial intelligence - it's essential not to stumble into jargon. It's a distinction I hope you've already come across, but when talking about large language models (LLM), it's crucial to differentiate the concept of language from the concept of thought.
To clear out the confusion, we need a different framework to think about LLMs, argue researchers at the University of Texas at Austin and Massachusetts Institute of Technology (MIT). In a paper titled “Dissociating language and thought in large language models: a cognitive perspective,” the researchers argue that to understand the power and limits of LLMs, we must separate “formal” from “functional” linguistic competence.
Read more on Tech Talks: you can find it here on Substack, too (
)